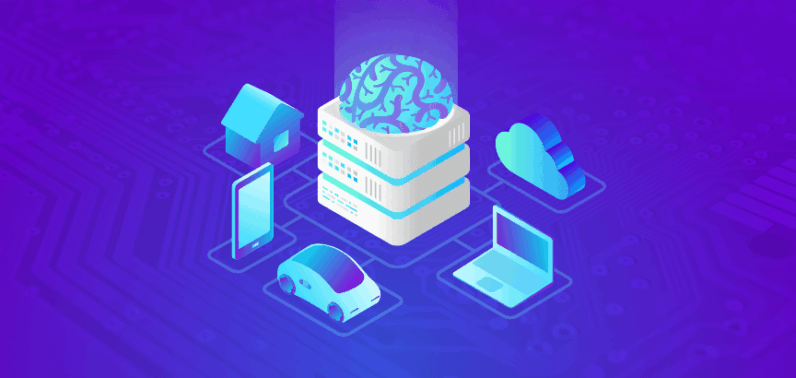
When we think about what AI is today and what it will offer in just five years, the difference is drastic. Today we have algorithms that recognize images and interactive voice applications. In five years, digital personal assistants will likely be the norm, digital research assistants will scan documents and compile relevant data, medical assistants will help diagnose and treat patients, financial modeling of “what if” scenarios will grow increasingly complex and accurate, and repetitive tasks will become automated in multiple industries. So, what will the AI transformation mean for your data center or IT infrastructure model?
Cloud or Data Center?
Today, many companies are using processing power in the cloud to develop AI applications, but is this always the most cost-effective way to implement AI in your organization? When it comes to predictable workloads, Michael Dell disagrees, “when you modernize and automate your on-premises and colocation systems, such workloads will cost less in your own data centers than in the public cloud.” According to Michael Miller from PC Magazine, Dell believes companies with cloud-first strategies will wake up and find that it was more expensive.
The cost of investing in the hardware needed for machine learning and deep learning is, like most hardware, a large capital investment up front, but in the long run, it is probably cheaper than paying a public cloud provider for hourly use of heavy processing power.
AI Hardware
The hardware needed for AI development today consists of AI-accelerating processors or neural network accelerators. Companies like Intel, IBM and Dell are developing hardware and software that makes it easier for companies to build their own AI systems and applications. For example, Dell is working with Intel to incorporate AI accelerators into their servers. Processors used for machine and deep learning today include:
GPUs – Because of their processing power, graphics processing units have been used for machine learning for several years. GPUs for AI has become a hot market for companies like Nvidia, with increasingly steep new competition to contend with.
FPGAs – Field-programmable gate arrays are integrated circuits containing logic blocks that can be configured by the purchaser post-manufacturing.
ASICs – Application specific integrated circuits are high-efficiency chips that are custom built for a particular device or application.
Companies like Google, Apple and Amazon have built their own customized hardware to carry out machine and deep learning tasks, forcing companies to work with them to take advantage of these new technologies. As companies begin to see the value in investing in their own hardware and data engineers,the hardware market for AI will take off.
What Does AI Mean for Your Data Center?
What does this processing power mean for the data center? – High density power and cooling. If you’re planning to invest in AI to improve efficiency or develop new products, make sure your data center can provide sufficient power and cooling to these racks. For many years, companies were using somewhere between 3 and 7 kW per rack. Today, data centers are being designed to support 36 kW per rack in anticipation or the density AI will require.
Data Foundry builds and operates high-density data centers that can support power and cooling requirements for up to 50 KW per rack. Underground infrastructure can tell you a lot about a data center’s capabilities. Learn more in our white paper.